Today, I want to tell you something about probability. Most people who hear this term intuitively understand what it means. If someone tells them there is a 90% chance for rain they know: better pack an umbrella today. Looking more closely, there are different definitions for probability in science and philosophy. In this blog post, I want to briefly introduce the two most common ones, which are also the two that frequently cause exhaustive discussions between their followers, and tell you about my pragmatic reasons to use one of the definitions.
About three centuries ago, there was an English Presbyterian minister called Thomas Bayes. It is not known how and why he spent a lot of time on probability theory, but he did. Only after his death, his most important work, nowadays known as the Bayes’ theorem, was made public by his friend Richard Price who sent Bayes’ work to the Royal Society accompanied with the following words:
‘I now send you an essay which I have found among the papers of our deceased friend Mr. Bayes, and which, in my opinion, has great merit, and well deserves to be preserved. Experimental philosophy, you will find, is nearly interested in the subject of it; and on this account there seems to be particular reason for thinking that a communication of it to the Royal Society cannot be improper. […]’
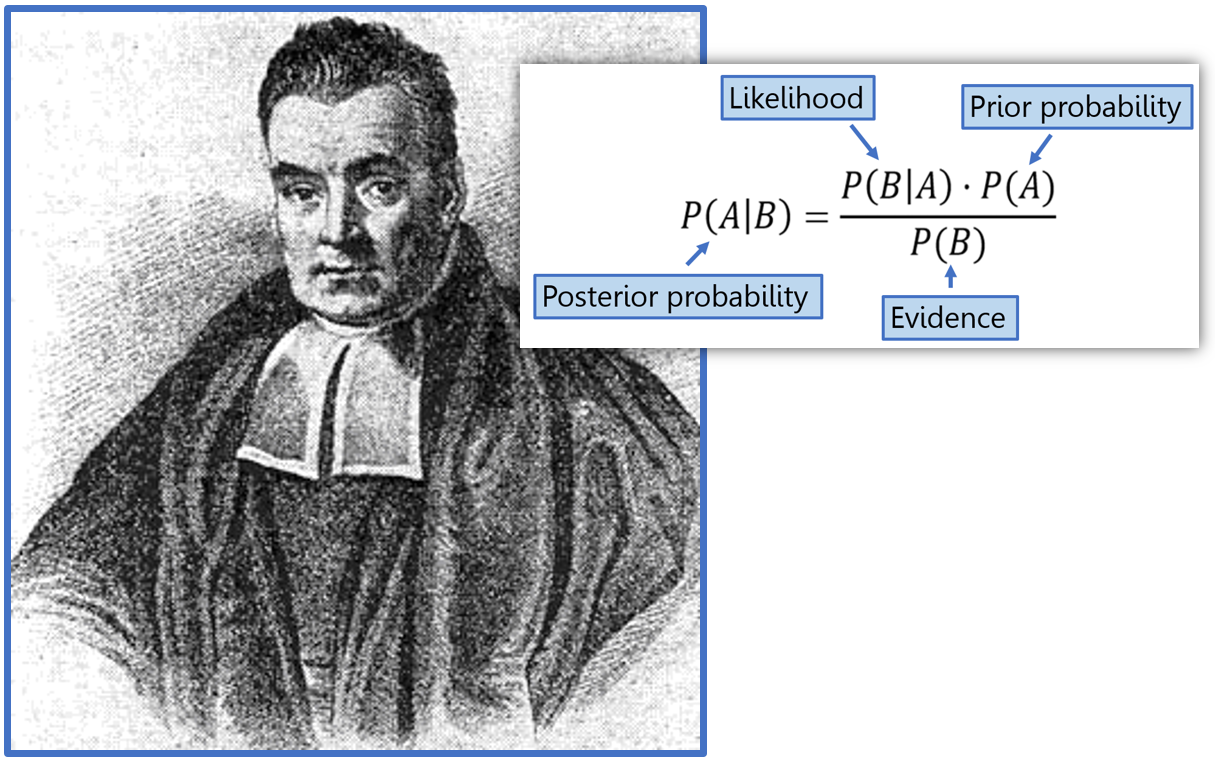
Figure 1: No one actually knows what Thomas Bayes looked like, but this is the picture that everyone uses; next to him you see his famous theorem.
If you are curious about the full essay, you can find it over here. Bayes never tried to publish his work himself. Of course, we do not know why exactly he did not try to do so, but rumor has it that he thought of his mathematical hobby as superfluous shenanigans.
Well, during his time, probability theory could seem somewhat marginal. Today, we actually need quite powerful computers to use his theorem. The theorem is considered in all kind of disciplines in which modeling is part. Examples are climate modeling and testing of new drugs. He inspired a whole new field in statistics, which to his honor later was called Bayesian statistics. In this field, people work with probabilities which they understand and interpret as reasonable expectations representing a state of knowledge, or as a quantification of personal belief (more in Jaynes, 1995).
Does everyone apply his method? No! There is a group of people, calling themselves frequentists, who refuse to model the Bayesian way. Why is that? Well, frequentists assume that there is such a thing as an objective probability (Jaynes, 1995). Only by repeating independent experiments over and over again, are we able to derive probabilities. This comes with the practical consequence that we can assign a probability only to repeatable random events, for example tossing a coin.
Frequentists and Bayesians represent two different schools of thought in defining and approaching probability. For many, many decades there have been philosophical discussions going on between the two groups. Some events are not repeatable and strict frequentists would therefore say that we cannot assign probabilities to them, think for example about the outcome of elections, the outcome of the football world championship, and the probability of a meteorite destroying the earth.
Another example would be assigning a probability to the sex of a baby (see Wolfgang Tschirk, 2014). The sex of a baby is already determined at the moment of fertilization. But, of course, it is not known to us and we also cannot immediately find out. A certain fertilization is a non-repeatable random event, but still we can think of a probability for expecting a baby boy. This means we need another concept of probability than the frequentists’ one to handle such issues. We already intuitively have an idea of the probability for having a male embryo without ‘hard data’ – such as from sonograms from this specific baby or any information on its parents. Knowing about the global sex ratios, that is 1.3:1.0 for male to female fertilization, we would state that a baby more probably is a boy.
In summary, frequentists and Bayesians have a different philosophical understanding of probability. Or, as some state even more clearly, they are talking about two different things that unfortunately have got the same name (e.g. stated by Rudolf Carnap).
For my research, probabilities play a large role in solving inverse problems (if you want to know more about inverse problems you can read my last post here). For modeling the subsurface, in my case landfills, it is necessary to give an initial idea about what the subsurface could look like. In classical inversions you simply give a starting model. Using a Bayesian approach, we can express this prior idea as a probability. Meaning that, instead of single numbers, we can use probability distributions as the starting model. In these models we can express our personal belief and/or quantify our expert knowledge about the investigated area. Of course, this means that we often have to let the computer run a lot, but finally we get rewarded with a result that is complemented with an uncertainty. In classical inversion, we would receive a single outcome satisfying the data, while there are often several possible solutions to the inverse problem satisfying the data equally well.
Uncertainty is valuable information. Especially for potential landfill mining projects where it can be – mildly speaking – quite tricky to find a single subsurface model that accurately represents the actual situation. Model parameters including uncertainty therefore provide interesting tools to help deciding if mining a certain landfill site makes economic sense or not.
Cited work:
Jaynes, E. T. (2003). Probability theory: The logic of science. Cambridge university press.
Tschirk, W. (2014). Statistik: Klassisch oder Bayes. Berlin, Heidelberg: Springer.
About the author:
![]() Christin is our ESR 1. She is German, has a master in geosciences from the RWTH Aachen University and has worked there for a few years as research assistant. Now she is doing her PhD research at the University of Ghent (Belgium) where she focuses on the modelling the landfill subsurface through integration of multi-sensor geophysical data. When she’s not planning on how to dig up your garbage, you might find her traveling, playing the piano, or smashing a volleybal! You can contact her via e-mail . |